Thermodynamics for allosteric modulation
SUPERVISOR: CHRIS OOSTENBRINK
Background.
Computer simulation of biomolecular complexes has developed into a powerful tool, complementary to experimental exploration (Karplus and McCammon, 2002; Van Gunsteren et al., 2006). By zooming in on the atomic level, complex biomolecular systems can be described at a time and space resolution often unattainable by experiments, thereby leading to significant insights into the molecular machinery of the cell.
One particularly challenging aspect in the function of proteins is allosteric modulation of their activity. In this project, we describe allosteric effects as changes in the function of the protein (ligand binding, enzymatic activity) due to modifications at a site that is not in close contact to the active, orthosteric, site. Such modifications could be the result of binding of an allosteric modulator, or the effect of a mutation at allosteric sites of the protein (Fenton, 2008; Carlson and Fenton, 2016). While original models suggested that allosteric effects were the result of conformational changes in the protein structures (Monod et al., 1965), in recent years these models have been generalized to more dynamic views. In general, the chemical equilibrium at the orthosteric site is shifted due to the allosteric site. In terms of statistical mechanics, this can be described as a shift of the conformational ensemble of microstates of the proteins. The population of the microstates changes, which may be observed as a conformational change of the orthosteric site, but could also lead to more subtle dynamic effects, that affect the activity of the protein (Kern and Zuiderweg, 2003; Ribeiro and Ortiz, 2016).
In molecular dynamics simulations, the conformational ensemble or microstates is explicitly simulated, offering excellent opportunities to study the mechanisms of allosteric effects. We have observed the dynamic effects of allosteric communication between two monomers of a nuclear receptor through molecular simulations (Venäläinen et al., 2010), and were also able to quantify the thermodynamics of the closely related effect of cooperativity in ligand binding (Bren et al., 2014).
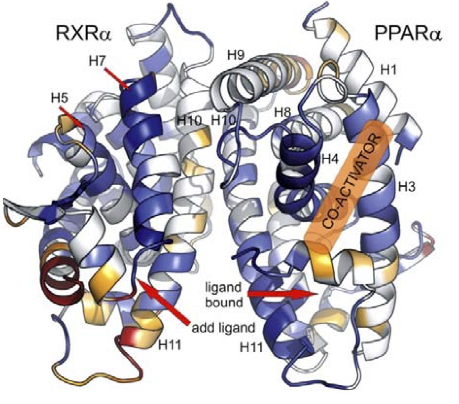
Furthermore, we were able to accurately predict binding free energies of a series of allosteric modulators to the ionotropic glutamate receptor (Nørholm et al., 2014). In general, free-energy calculations from molecular simulations are currently under much scrutiny by the pharmaceutical industry as a reliable means to rank candidates in lead optimization projects (Wang et al., 2015). However, traditional free-energy calculations that involve significant shifts in the populations of conformational ensembles often suffer from serious sampling issues as e.g. exemplified in (Graf et al., 2016). Most accurate free-energy methods make use of multiple, largely independent MD simulations. When significant shifts in the ensemble are to be expected, the equilibrium between different states needs to be adequately sampled in all individual simulations.
Aims and methods.
In this thesis we aim to develop methods to compute free-energy differences that involve significant allosteric effects. We hypothesise that shifts in conformational ensembles are best sampled by focusing the simulation effort in a single long simulation. Already in the calculations on the glutamate receptor (Nørholm et al., 2014), the one-step perturbation approach, which is based on a single simulation of a reference molecule was observed to outperform more traditional thermodynamic integration calculations. Similar conclusions were drawn for GTP as a model compound (Garate and Oostenbrink, 2013). In the one-step perturbation method a single intermediate state is simulated, which samples conformations that are relevant for multiple end-states. The main challenge in this method is the design of this, possibly unphysical, intermediate state (Oostenbrink, 2012). An optimal intermediate state leads to an ensemble that is a superposition of the constituting end-states, which is constructed in enveloping distribution sampling (EDS) method (Christ and van Gunsteren, 2007). Recently, we have refined EDS by modifying the functional form and significantly simplifying the associated parameter search (Perthold and Oostenbrink, 2018).
In this thesis, we will further develop the accelerated EDS method for application to allosteric effects. We will consider both the binding of allosteric modulators and the mutation of amino acids at allosteric sites. As one model system, we will consider changes in binding affinity of the Ab2/3H6 antibody, for which we have recently identified mutations in the framework region that improve binding to the complementarity determining regions, in collaboration with KUNERT (Margreitter et al., 2016; Schwaigerlehner et al., 2018). In collaboration with OBINGER, a second model protein will be the GTPase K-Ras, for which a mutant in the switch II region was recently reported, that leads to conformational changes in a remote switch I region, depending on the binding of a novel inhibitor protein or the substrate (Kauke et al., 2017). By performing extensive simulations of the EDS state of the system, possibly complemented with methods to enhance the conformational sampling of the entire protein, we do not only gain insight into the free-energy differences between the end states, but also in the concomitant shifts in the conformational ensembles and hence in the mechanism of allosteric communication.
Collaborations in this thesis involve KUNERT and OBINGER.
Bren, U., Fuchs, J. E., Oostenbrink, C. (2014) Cooperative binding of Aflatoxin B1 by Cytochrome P450 3A4: A computational study. Chem. Res. Toxicol. 27, 2136 -2147. doi: 10.1021/tx5004062
Carlson, G. M. and Fenton, A.W. (2016) What mutagenesis can and cannot reveal about allostery. Biophys. J. 110, 1912-1923. doi: 10.1016/j.bpj.2016.03.021
Christ, C.D. and van Gunsteren, W.F. (2007) Enveloping distribution sampling: a method to calculate free energy differences from a single simulation. J. Chem. Phys. 126, 184110. doi: 10.1063/1.2730508
Fenton, A.W. (2008) Allostery: an illustrated definition for the 'second secret of life'. Trends Biochem. Sci. 33, 420-425. doi: 10.1016/j.tibs.2008.05.009
Garate, J.A. and Oostenbrink, C. (2013) Free energy differences between states with different conformational ensembles. J. Comput. Chem. 34, 1398-1408. doi: 10.1002/jcc.2327
Graf, M.M.H., Maurer, M., Oostenbrink C. (2016) Free-energy calculations of residue mutations in a tripeptide using various methods to overcome inefficient sampling. J. Comput. Chem. 37, 2597-2605. doi: 10.1002/jcc.24488
Karplus, M. and McCammon, J.A. (2002) Molecular dynamics simulations of biomolecules. Nat. Struct. Biol. 9, 646-652. doi: 10.1038/nsb0902-646
Kauke, M.J, Traxlmayr, M.W., Parker, J.A., Kiefer, J.D., Knihtila, R., McGee, J., Verdine, G., Matthos, C., Wittrup, K.D. (2017) An engineered protein antagonist of K-Ras/B-Raf interaction. Sci. Rep. 7, 5831. doi: 10.1038/s41598-017-05889-7
Kern, D. and Zuiderweg, E.R.P. (2003) The role of dynamics in allosteric regulation. Curr. Opin. Struct. Biol. 13, 748-757. doi: 10.1016/j.sbi.2003.10.008
Margreitter, C., Mayrhofer, P., Kunert, R., Oostenbrink, C. (2016) Antibody humanization by molecular dynamics simulations - in-silico guided selection of critical backmutations. J. Mol. Recogn. 29, 266-275. doi: 10.1002/jmr.2527
Monod, J., Wyman, J., Changeux, J.P. (1965) On the nature of allosteric transitions: a plausible model. J. Mol. Biol. 12, 88-118. doi: 10.1016/S0022-2836(65)80285-6
Nørholm, A.B., Francotte, P., Goffin, E., Botez, I., Danober, L., Lestage, P., Pirotte, B., Kastrup, J.S., Olsen, L., Oostenbrink, C. (2014) Thermodynamic characterization of new positive allosteric modulators binding to the glutamate receptor A2 ligand-binding domain: combining experimental and computational methods unravels differences in driving forces. J. Chem. Inf. Model. 54, 3404-3416. doi: 10.1021/ci500559b
Oostenbrink, C. (2012) Free energy calculations from one-step perturbations. Methods Mol. Biol. 819, 487-499. doi: 10.1007/978-1-61779-465-0_28
Perthold, J.W. and Oostenbrink, C. (2018) Accelerating enveloping distribution sampling: enabling sampling of multiple end-states while preserving local energy minima. J. Phys. Chem. B, 122, 5030 - 5037. doi: 10.1021/acs.jpcb.8b02725
Ribeiro, A.A.S.T. and Ortiz, V. (2016) A chemical perspective on allostery. Chem. Rev. 116, 6488-6502. doi: 10.1021/acs.chemrev.5b00543
Schwaigerlehner, L., Pechlaner, M., Mayrhofer, P., Oostenbrink, C., Kunert, R. (2018) Lessons learned from merging wet lab experiments with molecular simulations to improve mAb humanization. Protein Eng. Des. Sel., online. doi: 10.1093/protein/gzy009
van Gunsteren, W.F., Bakowies, D., Baron, R., Chandrasekhar, I., Christen, M., Daura, X., Gee, P., Geerke, D., Glättli, A., Hünenberger, P.H., Kastenholz, M.A., Oostenbrink, C., Schenk, M., Trzesniak, D., van der Vegt, N.F.A., Yu, H.B. (2006) Biomolecular modeling: goals, problems, perspectives. Angew. Chem. Int. Ed. 45, 4064-4092. doi: 10.1002/ anie.200502655
Venäläinen, T., Molnar, F., Oostenbrink, C., Carlberg, C., Peräkylä M (2010) Molecular mechanism of allosteric communication in the human PPARalpha-RXRalpha heterodimer. Proteins 78, 873-887. doi: 10.1002/prot.22613
Wang, L., Wu, Y., Deng, Y., Kim, B., Pierce, L., Krilov, G., Lupyan, D., Robinson, S., Dahlgren, M.K., Greenwood, J.R., Romero, D.L., Masse, C., Knight, J.L., Steinbrecher, T., Beuming, T., Damm, W., Harder, E., Sherman, W., Brewer, M., Wester, R., Murcko, M., Freye, L., Farid, R., Lin, T., Mobley, D.L., Jorgensen, W.L., Berne, B.J., Friesner, R.A., Abel, R. (2015) Accurate and reliable prediction of relative ligand binding potency in prospective drug discovery by way of a modern free-energy calculation protocol and force field. J. Am. Chem. Soc. 137, 2695-2703. doi: 10.1021/ja512751q