Multi-omics analysis of CHO cell lines
SUPERVISOR: Nicole Borth
Background.
Over the last 10 years, our group and others have collected big data on several levels of cellular
information across a wide array of CHO cell lines, including genome sequences of multiple cell lines,
epigenetic marks such as DNA-methylation and histone modifications, transcriptome and proteome
analysis. Few studies have been comprehensive in the sense that all of these were analysed from the same
experiment so that a full integration of multiple layers of cellular integration would be possible. In
addition, in most of these experiments the generated data set only from this experiment was analysed
without a global view across the many different CHO cell lineages and subclones.
For example, the typical situation for a transcriptome analysis is that a handful of cell lines or culture
conditions/culture states are being compared to look for genes or pathways that are differentially
expressed reflecting the specific situation of the cell in one of the investigated conditions or connected to
a behavior of interest, such as high productivity. Given the high variation of different CHO cell lineages
and subclones and the variation in transcriptomes that ensues due to culture, process or medium changes,
the results are always limited to the specific cell line or condition investigated and thus cannot be
universally applied or extrapolated. In addition, even for the same purpose and under the same condition,
most cellular pathways are redundant – that is, there are more ways to achieve the same goal and cells
are able to use all of them. Therefore, most studies that investigated genes related to increased cellular
productivity identified similar pathways as affected, but rarely the same gene(s), further complicated by
the fact that the precise expression level of multiple genes may be involved in determining phenotypes,
not just which genes are turned on or off. Just as in the Magic Eye 3D image below, the patterns that
underlie cellular properties will remain hidden amongst the complexity of the available data sets and will
require innovative thinking and tools to be made visible.
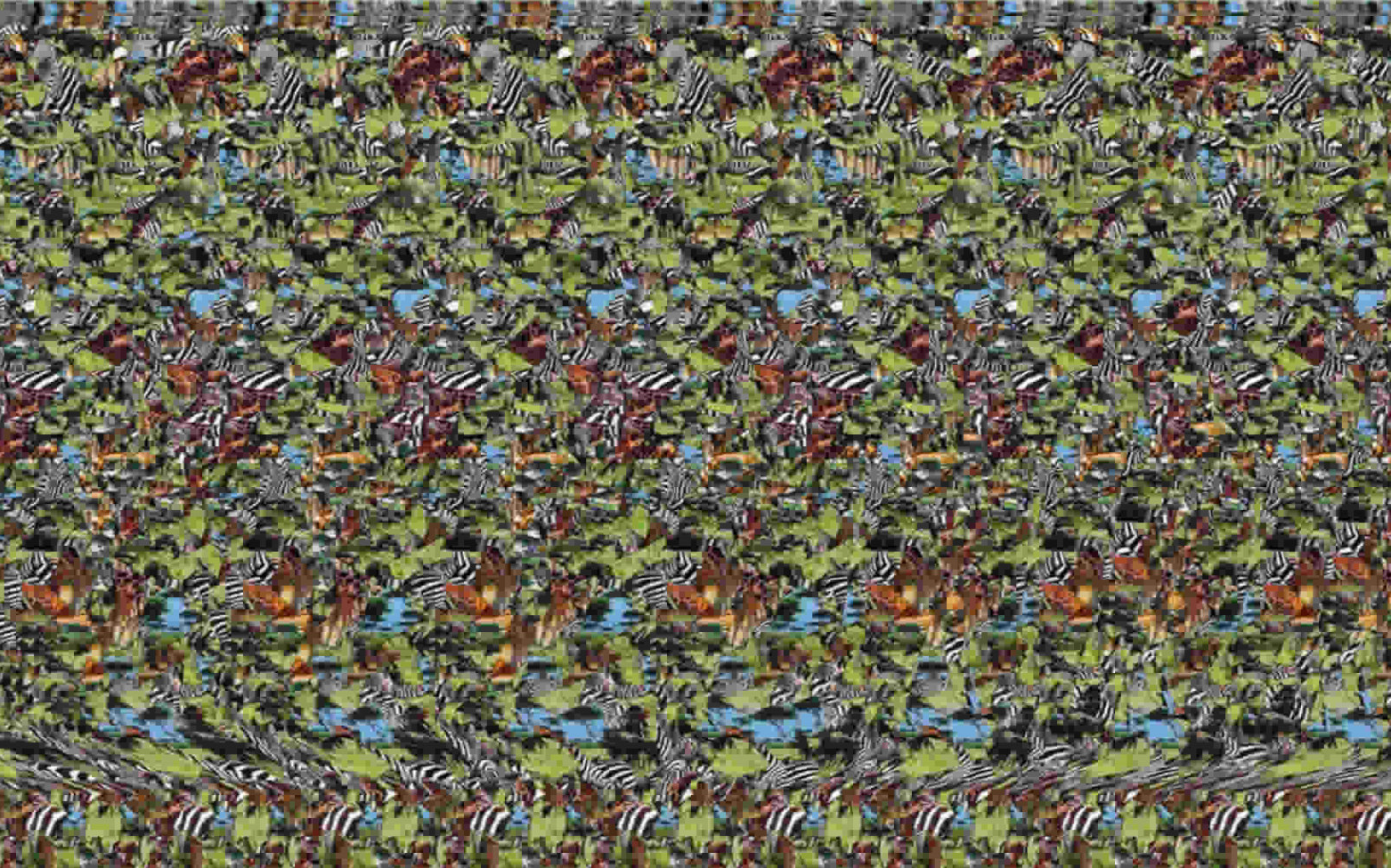
Aims and methods.
The main objectives of this project are:
- To obtain a comprehensive overview over both coding and non-coding genes expressed across the variety of CHO cell lines and lineages using all in-house and publicly available datasets
- To develop new analysis pipelines using either a combination of available scripts and programs or writing new ones, that integrate multiple layers of regulation, for instance by connecting
- DNA-methylation patterns with the resulting transcriptome or the transcriptome and miRNome with the resulting proteome
Currently genome sequences for >20 cell lines area available, DNA-methylation patterns for 12 cell
lines/states, approx. 400 transcriptome data sets including coding and long-noncoding RNAs and
proteome data for 20 samples. Approximately two thirds of these data sets were generated in house, so
we also have detailed information about cell behavior and process performance. We also have a
comprehensive dataset for an industrial fedbatch process including all of the above analyses plus detailed
metabolic characterization (generated in collaboration within the eCHO systems ITN network).
Thus, we can now aim to apply existing and/or develop new pipelines for analysis of big data sets such as
these, to extract seemingly hidden players and complex interactions between genes that control relevant
cellular properties and stress responses, so as to identify new engineering targets or to control cellular
behavior for improved process performance. For instance, the majority of highly expressed genes seems
to be expressed across all samples so far investigated, however at different expression levels. Thus it
seems that the diversity in phenotypes observed in CHO cells is mainly driven by the individual pattern of
expression levels across this set of genes, rather than by new genes being turned on or off. This has
important implications in the design of engineering strategies as it would entail being able to control
multiple genes in a fined tuned and individually adjusted manner, rather than the crude overexpression
of one gene so far employed.
Further, the rules and connections between the different layers of regulation have not been investigated
in full detail yet, due to the lack of comprehensive studies that collect complete datasets (understandably
due to the cost of such endeavours). Given the rich datasets available in our team we can start and
implement the first steps and pipelines for such an integrated type of analysis.